Numerical Solutions of Differential Equations using Artificial Neural Networks
DOI:
https://doi.org/10.14295/vetor.v31i2.13793Keywords:
Neural networks, Differential equations, OptimizationAbstract
In this article, we study a way to numerically solve differential equations using neural networks. Basically, we rewrite the differential equation as an optimization problem, where the parameters related to the neural network are optimized. The proposal of this work constitutes a variation of the formulation introduced by Lagaris et al. [1], differing mainly in the form of the construction of the approximate solution. Although we only deal with first and second order ordinary differential equations, the numerical results show the efficiency of the proposed method. Furthermore, this method has a great potential, due to the amount of differential operators and applications in which it can be used.
Downloads
References
I. Lagaris, A. Likas, and D. Fotiadis, “Artificial neural networks for solving ordinary and partial differential equations,” IEEE Transactions on Neural Networks, vol. 9, no. 5, pp. 987–1000, 1998. Available at: https://doi.org/10.1109/72.712178
C. Aggarwal, Linear Algebra and Optimization for Machine Learning, 1st ed. New York, USA: Springer, 2020.
G. Strang, Linear Algebra and Learning from Data, 1st ed. Massachussets, USA: Cambridge Press, 2019.
O. I. Abiodun, A. Jantan, A. E. Omolara, K. V. Dada, N. AbdElatif, and H. Arshad, “State-of-the-art in artificial neural network applications: A survey,” Heliyon, vol. 4, no. 11, p. e00938, 2018. Available at: https://doi.org/10.1016/j.heliyon.2018.e00938
G. Cybenko, “Approximations by superpositions of a sigmoidal function,” Mathematics of Control, Signals and Systems, vol. 2, no. 4, pp. 303–314, 1989. Available at: https://doi.org/10.1007/BF02551274
K. Hornik, M. Stinchcombe, and H. White, “Multi-layer feedforward networks are universal approximators,” Neural Networks, vol. 2, no. 5, pp. 359–366, 1989. Available at: https://doi.org/10.1016/0893-6080(89)90020-8
R. Khemchandani, A. Karpatne, and S. Chandra, “Twin support vector regression for the simultaneous learning of a function and its derivatives,” International Journal of Machine Learning and Cybernetics, vol. 4, pp. 51–63, 2013. Available at: https://doi.org/10.1007/s13042-012-0072-1
V. Avrutskiy, “Enhancing function approximation abilities of neural networks by training derivatives,” IEEE Transactions on Neural Networks and Learning Systems, vol. 32, no. 2, pp. 916–924, 2021. Available at: https://doi.org/10.1109/TNNLS.2020.2979706
L. Evans, Partial Differential Equations, 2nd ed. Providence, USA: American Mathematical Society, 2010.
R. Byrd, J. C. Gilbert, and J. Nocedal, “A trust region method based on interior point techniques for nonlinear programming,” Mathematical Programming, vol. 89, no. 1, pp. 149–185, 2000. Available at: https://doi.org/10.1007/PL00011391
J. Herskovits, “A view on nonlinear optimization,” in Advances in Structural Optimization: Solid Mechanics and Its Applications, 1st ed. Dordrecht, Netherlands: Springer, 1995, vol. 25, pp. 71–116.
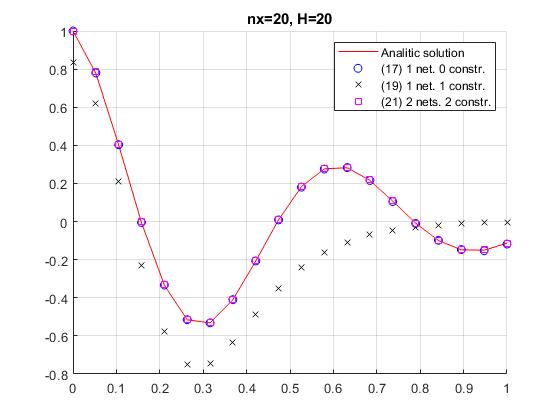